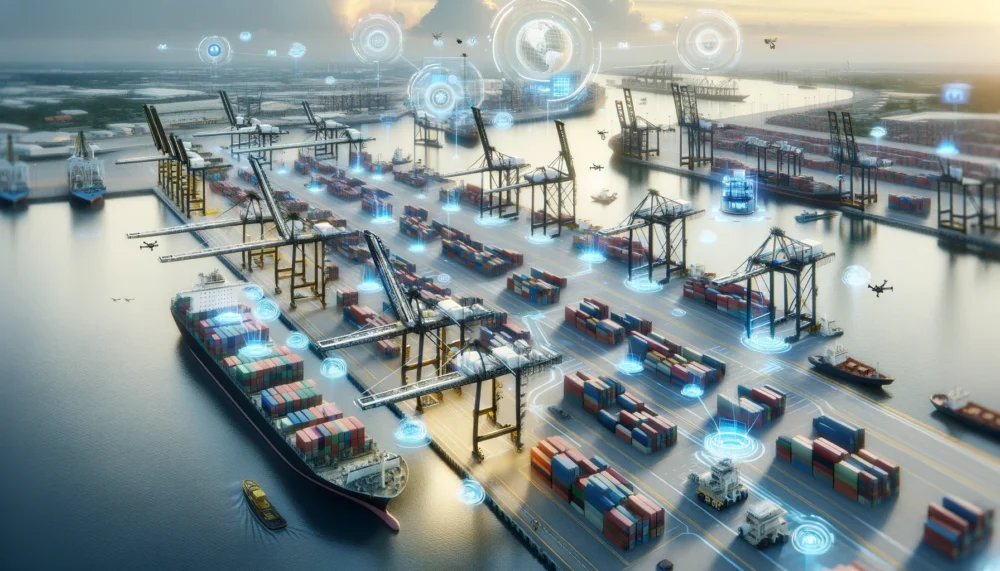
A quick start to AI tools and their applications in ports
Artificial Intelligence is having a big impact on different activities... and maritime and port sector is no exception. Here is a clear view on important topics related to AI and its applications in the maritime sector. An article written by a human person!
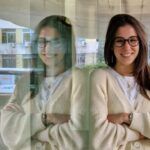
Chiara Saragani is a researcher at CENIT and PhD student in digitalization at the Port of Barcelona.
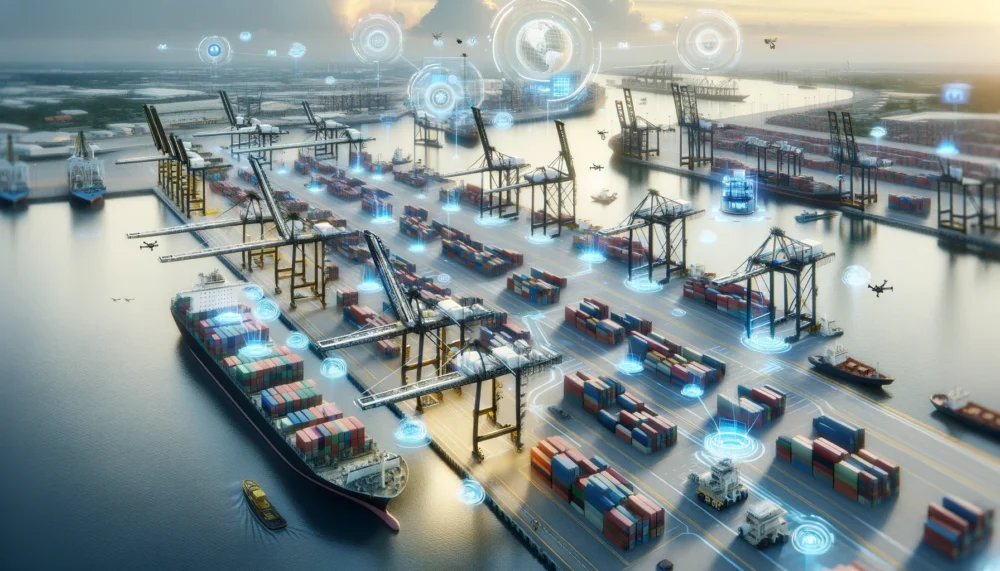
Artificial intelligence and all its facets
Artificial Intelligence (AI) is the science that permits machines to perform human functions like learning, solving problems, analysing information, creating videos and images and so on. It can be combined with other technologies, and it can help humans improve their activities due to its capacity to analyse big amounts of data and get results fast.
Investments in AI have grown rapidly in the last decade. The following bar chart explains the annual private investment in artificial intelligence.
From Machine Learning to Deep Learning
Artificial Intelligence can be weak or strong. Weak AI – also said Narrow Artificial Intelligence – elaborates results for specific tasks. Examples of it are face recognition or an internet search. On the other hand, strong AI – also said Artificial General Intelligence – can combine different activities and are really close to a faithful representation of human activities. Currently, Artificial General Intelligence solutions do not exist.
Artificial Intelligence is composed of different categories, due to the fact that it is a wide concept. Within this scope, one of the most important approaches is Machine Learning (ML), which uses large data sets to detect patterns and, consequently, synthetise and predict information. Machine Learning requires a minimum of human interference while it is generating results.
One of the best-known branches of ML is Deep Learning (DL). This sub-discipline uses artificial neural networks in order to produce a huge number of iterations to create more accurate results, with less human intervention compared to the broader scope of Machine Learning. Neural networks derive their name from the fact that they try to simulate the neurons of human brains to obtain results.
Based on what has been previously written, Deep Learning processes vast amounts of data as inputs, which are interconnected across different layers of nodes. These nodes interact in order to produce different outputs, which are iterated several times.
The following figure shows graphically the big difference between Machine Learning and Deep Learning.
Generative AI
A more specific branch within Deep Learning is Generative AI, which uses large neural networks, also called Large Language Models (LLM), to not only collect and analyse data, but also create text, videos, images, and new information. Generative AI is the most complex concept in the AI field, and it also has the most impact.
Indeed, with the development of large language models like Chat GPT, Perplexity, Google Gemini and so on, for the first time a new technology with extreme levels of complexity does not require a base level of computing expertise from end users.
To sum up, it is possible to affirm that Artificial Intelligence could achieve multiple functions in the future. It will be able to interact with humans thanks to its possible capabilities. In order to provide insights, the following chart represents some of the possible skills of Artificial Intelligence compared with human performances. As a starting point, the AI capabilities are normalized to an initial performance of -100. When they reach a score of 0, they become comparable to human performance, and beyond that point, they can even overtake human abilities.
Some of these AI capabilities - like reading comprehension, image recognition and language understanding - have already achieved a positive score. On the other hand, some of them, like complex reasoning and math-problem solving, are still under human performance.
Possible applications of artificial intelligence for the maritime sector
Looking at the shipping sector, AI models can be helpful in many ways. First of all, they can be a perfect support for ship planning, by simulating possible scenarios to understand the best dimensions of stern, prow, and other factors. Moreover, it could be used for fleet management and optimization, helping to select the best route for navigation, thereby reducing costs. Finally, another possible use case could be an alert system for reducing the probability of incidents, not only at sea but also within a port's boundaries.
From the point of view of the Port Authority, AI applications can be divided into different topics:
- Supervision and monitoring of the port area. AI systems can be used to monitor almost everything. For example, they can be applied to cargo detection and product movements, helping to monitor what is in the port and where. Better control of such objects can lead to better management of them.
- Automated alert systems. AI algorithms are able to understand when an accident is occurring, helping the port security system to be more proactive in managing it. Alert systems can supervise not only possible incidents at sea, but also those between trucks, cars, etc.
- Optimization of port logistics activities. New AI systems can help decision-makers optimize track flow entering and exiting the port. They can also be useful in allocating better port resources with the aim of minimising costs and effort.
- Generative AI for port employees. As different companies have already discussed, Generative AI will be implemented in the future in the daily lives of employees. These systems will support them in their activities. An example of this is Chat Copilot M365, which is a generative model in the Microsoft system. This tool can be used for Teams, Outlook, PowerPoint, Word and Excel. It can summarise contents, create presentations and graphics, create replies to email and so on.
- Infrastructure monitoring. AI can help to support predictive maintenance in order to reduce risks. It is able to detect possible incidents in infrastructure and manage them better.
- Autonomous ships. Autonomous ships can work with the use of AI. They can be used in the future to support maritime activities. This can be extended to other means of transport like trucks or terminal machinery.
- Data management. AI can be useful for two different objectives. Firstly, it can provide support to the cybersecurity system, to make it more resilient and able to detect possible attacks. It can also be useful for data storage, to optimise database capacity. Finally, collection of data regarding the port calls can be useful to improve ETA values and, as consequence, optimize the logistics of the port.
The following figure shows the annual private investment done annually by European Union and UK, China and US, by focus area.
The european commission stated its opinion through the AI Act
On 13th of March 2024, the European Parliament approved the AI Act. This legislation aims to ensure trust in AI while reducing the risks that can result from certain AI systems. It establishes specific requirements and obligations for developers and deployers of AI products.
As reported on the official website of the European Union, the proposed rules will:
- address risks specifically created by AI applications;
- prohibit AI practices that pose unacceptable risks;
- determine a list of high-risk applications;
- set clear requirements for AI systems for high-risk applications;
- define specific obligations for deployers and providers of high-risk AI applications;
- require a conformity assessment before a given AI system is put into service or placed on the market;
- put enforcement in place after a given AI system is placed on the market;
- establish a governance structure at European and national level.
The Regulatory framework
The Regulatory framework is composed of 4 levels of risk for AI systems:
- Unacceptable risks are those that can threaten fundamental rights or social values and can lead to serious incidents. Such types of AI applications are prohibited. They include all activities connected to facial recognition, those that can influence children’s behaviors, and that can classify people according to their status or personal characteristics.
- High risks include systems that are developed in products which fall under the conditions of EU product safety legislation, and those that are involved in specific areas like education, migration and so on. These applications will be evaluated not only before their launch on the market, but also during their lifespan.
- Limited risks are linked to the risks associated with the lack of transparency in AI usage. The European Commission will introduce specific transparency rules to inform companies beforehand. These include applications that involve interaction between humans and machines.
- Minimal or no risks are applications like the spam filters. The EU stated that the vast majority of AI systems in Europe are under this category.
A series of steps are being proposed to manage particularly high-risk applications.
Moreover, the European Commission stated that large language models like ChatGPT are not classified as high risk, but they will have to comply with European legislation on transparency requirements and EU copyright law.
Is AI aware of the environmental challenge?
One of the common topics that is arising from AI is that, despite all the benefits that it brings us, it requires a lot of electricity. This is because AI needs to process a huge amount of data all the time, requiring all the necessary components to use electricity to work.
Lyline Lim, Head of Impact and Sustainability at PhotoRoom, in an article published in Forbes, stated that an important factor in consumption is when AI makes an inference. An inference is the process by which AI answers a user’s query. Each inference requires a specific amount of GPU processing power, so the more queries there are, the more energy is consumed.
Moreover, another important topic is energy consumption during model training. Naturally, the training time is directly proportional to the complexity of the model itself. During this time, the model consumes energy.
In addition, the following chart shows the computation used to train notable AI systems, categorized by the affiliation of researchers. Note that the values are expressed in petaFLOP, which is the unit of measurement for this type of information.
Optimize energy consumption
To put this into numbers, the article published in The Verge stated that training a model like Chat GPT-3 uses almost 1,300 megawatt hours of electricity. This is more or less equivalent to the consumption of 130 US homes.
Different solutions are already being developed in the world in order to address to this problem. Some of the alternatives could be the use of efficient algorithms, which optimize energy consume, in order to reduce peaks of it.
One of the solutions which could be easy implemented is a better balance between the activities run during the day (when it is more possible to use sustainable resources) and during the night, or also managing the activities with respect to the different seasons.
Finally, another solution could be the use of green energy-efficient hardware or green clouds . They have a specific design that helps to reduce the energy consumption.
To conclude, Artificial Intelligence stands at the precipice of transformative change. Its potential to revolutionize industries, enhance human capabilities, and solve complex problems is undeniable.
However, with great power comes great responsibility.
As we adopt AI, it’s crucial to ensure its responsible implementation in accordance with ethical standards and protective measures.