WHAT IS MACHINE LEARNING?
Machine learning is a key technology to drive the digitalization of the sector. Although its emergence occurred four or five years ago, the advent of the Internet of Things (IoT), 5G and Edge Computing represent a substantial improvement in the collection, transport and transmission of data obtained from sensors, cameras and other information-gathering devices.
As its name suggests, machine learning consists of “teaching” machines to learn by themselves so that their work is optimized and are able to distinguish what data is or is not useful. If we make a comparison with the human brain, the devices that capture the information are the equivalent of the senses: they generate a series of data that is processed and organized from an algorithm, which is gaining in precision as it is trained with simulations and accumulates more data from the sensors. Thus, it completes its learning and improves its functionality.
Machine learning is part of our lives: it auto-completes sentences when we write an email and unlocks our mobile phone thanks to face detection.
“The machine learns to discern on the basis of repetition because it has seen a million different cases, not because it is intelligent. People will ultimately make the decisions supported by the machines,” explains Lutz Kretschmann, head of the Maritime Operations Management Team at Fraunhofer CML and co-author of the report 'Machine Learning in Maritime Logistics'.
The German institute report analyzes the evolution of this technology in the maritime supply chain and highlights the application of various use cases that demonstrate its ability to optimize certain logistics processes.
WHAT ARE THE BENEFITS OF MACHINE LEARNING IN PORT OPERATIONS?
David Serral, director of the Information Systems Department of the Port of Barcelona, points out that it is the ports with the highest volumes of traffic such as Rotterdam, Hamburg, Los Angeles, Qingdao or Barcelona, which are leading their implementation based on pilots and identifies the processes that can benefit from the potential of machine learning: “the automation and improvement of certain daily operations, such as the movement of containers and the reduction of waiting time for trucks that access port facilities. Regarding infraestructures, predictive models can help forecast traffic volume growth in order to adapt appropriately,” he exemplifies.
In more general terms, Kretschmann explains that the application of this technology is perfect for maritime logistics as the industry is "extremely rich in data generation.”
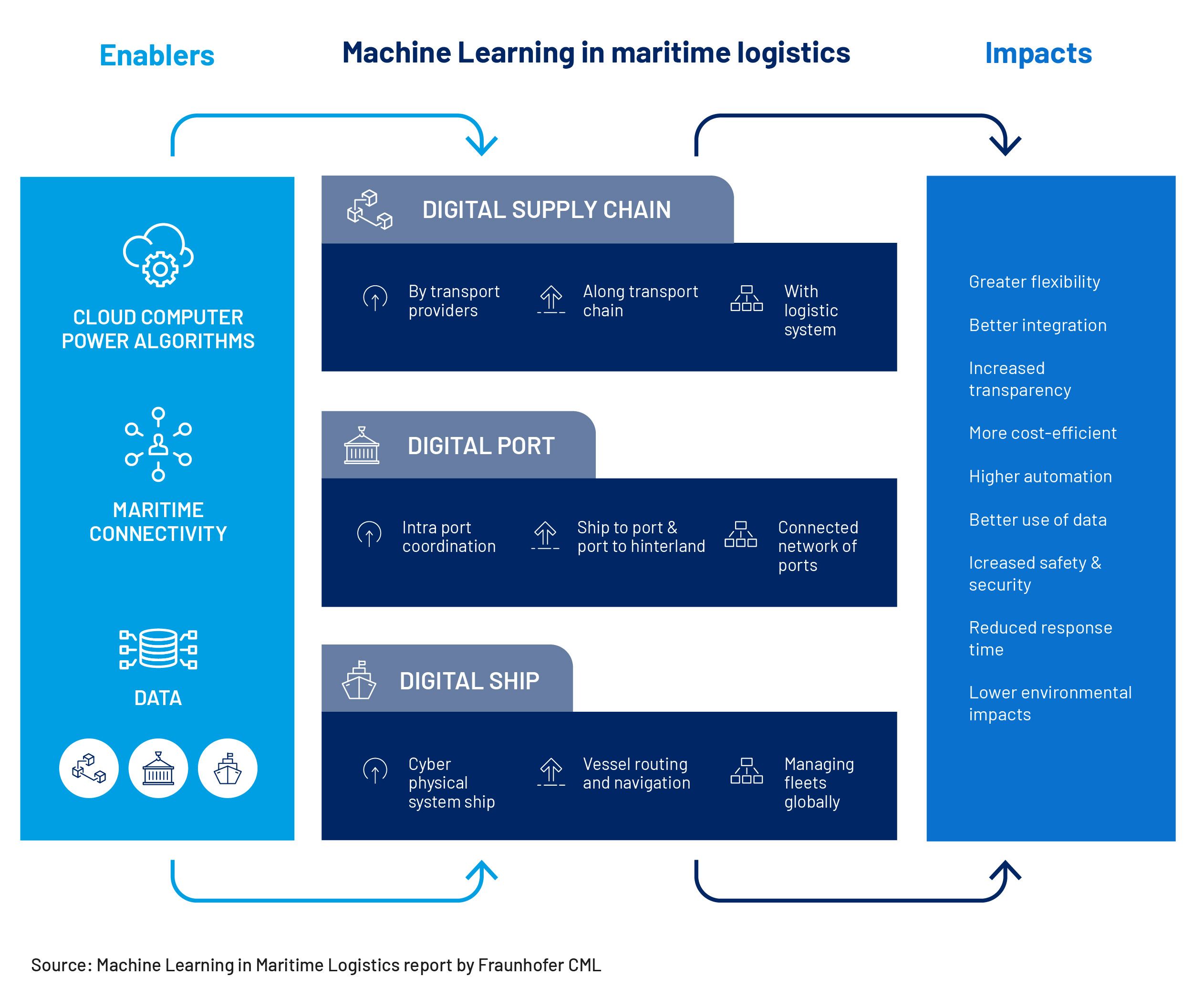
IN WHAT LOGISTICS-PORT AREAS HAS IT BEEN APPLIED SO FAR?
According to the Fraunhofer CML report, the spearhead of the introduction of machine learning in the sector has been the obligation to equip ships with Automatic Identification Systems (AIS), the ship tracking system that allows its unique identification, as well as its position, course and speed.
The information stored by the AIS systems possibilitated the creation of a database with the navigation patterns of a merchant fleet as a whole and of the individual behavior of a vessel. This information enhances possible use cases, such as the forecast of traffic density and frequency, risk scenarios in restricted waters or the detection of abnormal behaviors that help avoid collisions.
HOW HAS IT BEEN EXECUTED?
Fraunhofer CM has developed several proofs of concept explained in detail in the report. The first one is based on the arrival time of the ships at the main German ports, since the general lack of punctuality negatively impacts the planning capacity of the supply chain.
To improve its prediction, a series of algorithms were established from the correlation of between 3 and 4 million records generated daily by the AIS system with meteorological and hydrographic parameters, which have made it possible to establish a forecast up to 72 hours in advance, with a margin of error of approximately 3 hours.
Another use case is associated with the assessment and reduction of emissions from ships. To define this model, Fraunhofer CM used two data sets: those provided by the AIS (which includes the movement, size and speeds of ships) and environmental data (ocean conditions and meteorological information).
Normally, information on the consumption of the main engine and the emissions generated by ships on the high seas is not available, so an estimation model was used in combination with a clustering algorithm, which groups a series of vectors according to a determined standard to be able to identify classes of ships with similar characteristics and thus estimate these values.
Subsequently, opportunities to reduce emissions were identified with the help of an optimization model that suggests more efficient alternative routes.